Rochester, New York – A group of researchers at the University of Rochester introduced a machine-learning algorithm they developed. This machine can spot tweets posted under the influence of alcohol. This technology, they said, could be used to better understand the frequency and settings of alcohol consumption, and maybe even help the public respond to and prevent health issues.
The group of researchers developed two technologies. The first is a way to train a machine-learning algorithm to spot tweets that relate to alcohol and those sent by people drinking alcohol at the time. The second is a way to find a Twitter user’s location to determine whether they are drinking at home or not.
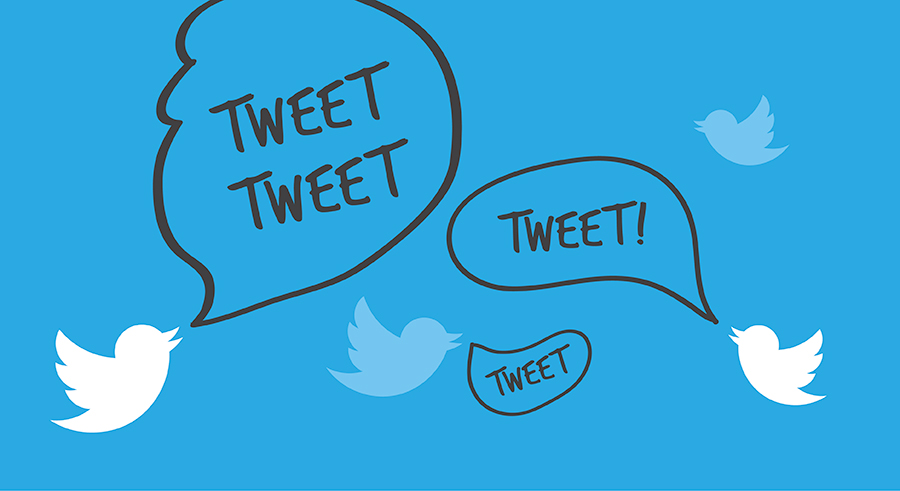
Process to find ‘drunk tweets’
The group led by Nabil Hossain collected thousands of tweets posted from July 2013 to July 2014 in New York. Then, they filtered messages that mention alcohol or drinking-related words like “drunk,” “beer,” and “party.”
Researchers then passed the 11,000 tweets resulting through three human operatives on Amazon’s Mechanical Turk crowdsourcing platform. They were asked several questions such as: Does the tweet make any reference to drinking alcoholic beverages? If so, is the tweet about the tweeter him or herself drinking alcoholic beverages?
“Our future work will perform a comprehensive study of alcohol consumption in social media around features such as user demographics, settings people go to drink-and-tweet. We can explore the social network of drinkers to find out how social interactions and peer pressure in social media influence the tendency to reference drinking,” researchers wrote in the paper.
One step further
If just finding out if the tweet was written under the influence of alcohol is not enough, the team also tried to find out whether the drunk-tweeters were at home or somewhere else when posting.In
In order to identify a Twitter-geolocated place, they put together a list of words people are likely to use when they are home, including “bath,” “sofa,” “TV,” “sleep,” and “home” and filtered thousands of tweets accordingly.
Again, they asked twitters to establish whether the tweets had been sent from somebody’s home, and then honed the resulting dataset with other information such as the location of the last tweet of the day. This way, they created another algorithm they say can determine a user’s home location with an accuracy of up to 80%.
Source: PC Mag
When you yourself have a serious drinking problem, its most likely necessary to be detoxified in-patient at a hospital or treatment center.As you begin to feel better, and you WILL,with elp, and commence to eat normally, and rest ordinarily, they are going to then start to wean you off the medicines, so that you will then be properly alccohol and drug free.
there are a number of books to show you how this is a great one check it out! quitdrinkingtoday2016.blogspot.ba
Thanks for finally talking about alcoholism
AA has shown to own a relatively low success rate. However you should try to incorporate amino acids into the diet. That has shown to decrease cravings. The reason is because the brain requires it is dopamine boost it gets from liquor. Once you quit your brain neurotransmitters are diminished leading to cravings and urges that are difficult to ignore. Anyway, alcoholism can be cured however you should treat the desire, definitely not the behavior.
These day there are techniques to naturally reverse alcoholism. You don’t have to simply take the pills or shots. Check out this brand treatment that is new taking a quick look on google for John Dawkins stop drinking program to get this method.
Also, thank you for allowing for me to comment!